

For example, there are multiple studies in the literature searching potential metal organic framework (MOF) platforms for carbon capture (with various degrees of rigor for an early example, see Fernandez et al. While AI/ML/BD approaches can provide (and “learn”) hidden relationships and mechanisms that experimental approaches may overlook, current projects/efforts are still limited to a narrow definition of the problem and parametric space. However, the parameter space is still intractable. The digital approach enables efficient mapping across variables as well as data generation. In materials, in simplest terms, the majority of researchers are utilizing AI/ML/BD approaches to efficiently explore the parametric space of materials design and/or discovery. Paraphrasing Jason Hattrick-Simpers from the Acceleration Conference, “useful models are useful, but not all models are useful.”
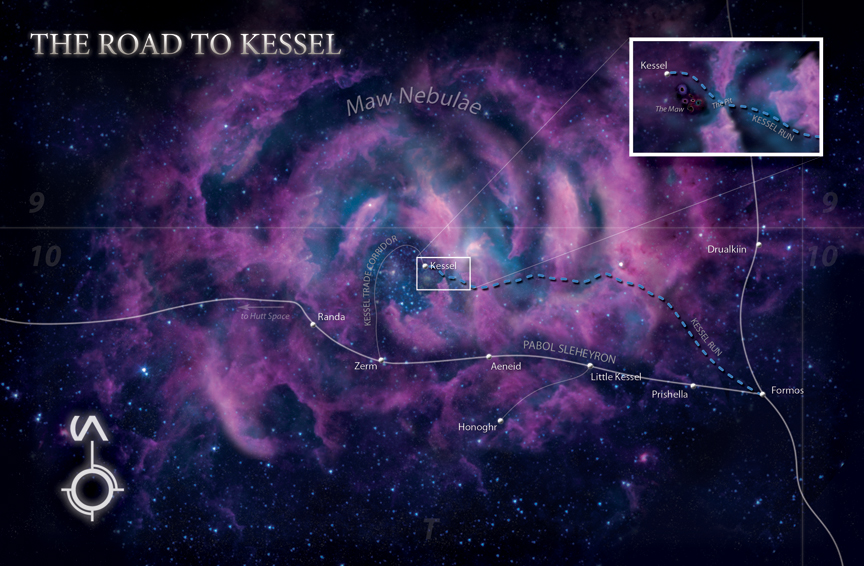
But it is important to reflect on the field before diving headfirst into the hype and adding AI and ML to every research project. It’s a kind of simulated microscopy able to explore the multidimensional space of “data.” Spooky stuff.Ĭlearly there is excitement in the field, and we may very well be at the cusp (or in the midst) of an unprecedented Kuhnian shift. Modern data science is more open-ended-we don’t know a priori the key variables or the output-the AI/ML algorithms discover new rules, principles, and mechanisms within the data, via variables and patterns either overlooked or undetectable by other means. Where data science diverges from traditional computational science is essentially in the control of variables-prior computational methods were more akin to simulated experiments, directed by input control parameters with a desired/predicted response. 2, 3 In the past decade, data science (and its related computational approaches) has been labeled the fourth paradigm of scientific discovery, 4 where paradigms one to three are (1) empirical evidence, (2) scientific theory, and (3) computational science.
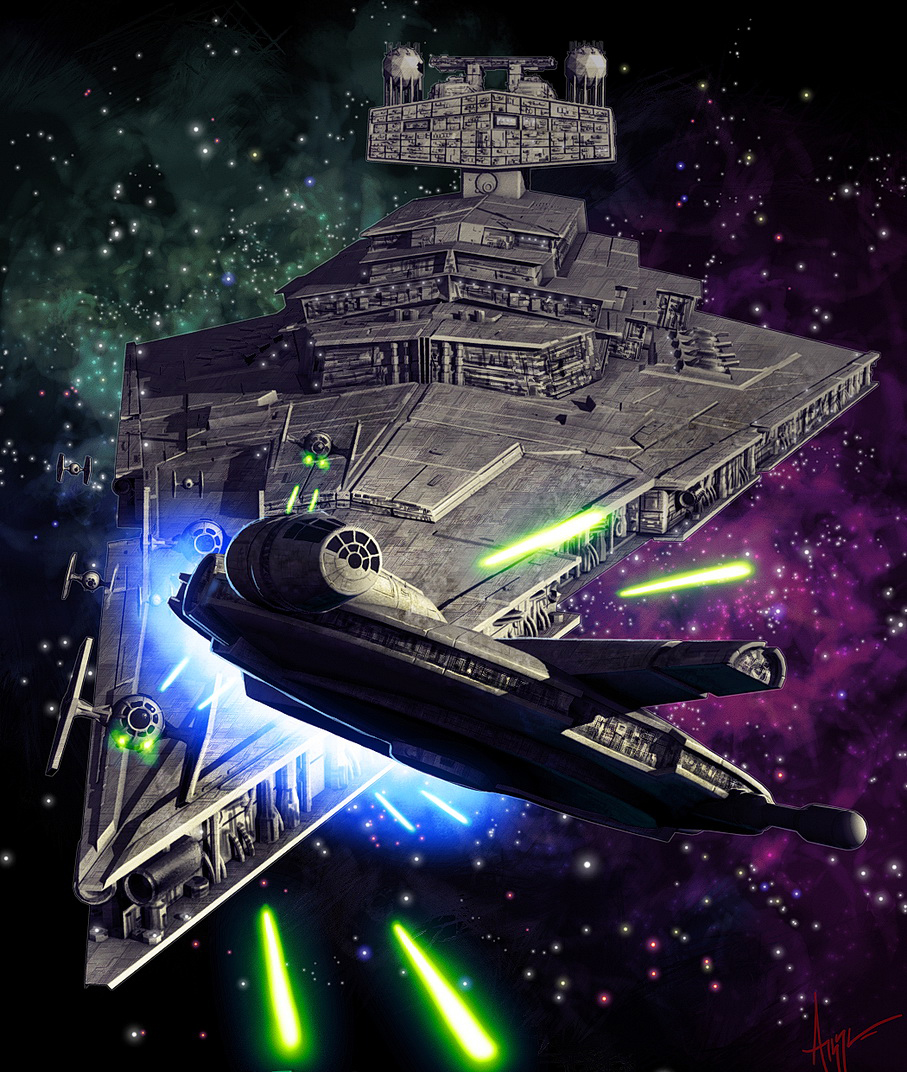
Indeed, AI, ML, and so-called big data (BD) approaches are the means du jour across modern research and development, from fintech to healthcare to self-driving cars as well as physical sciences such as chemistry and materials. With such a jam-packed and demanding schedule digesting a large variety of information, it was only afterwards I was able to reflect on the event and its potential in the broader scope of materials in general. Also, some time for Paloma cocktails and salsa dancing. Sessions ranged from technical (e.g., Methods in Machine Learning, Data Analysis, and Modeling) to applied (e.g., Discoveries in Energy), to engaging panel discussions (e.g., How to Commercialize Materials-on-Demand). The goal was clear: bespoke materials solutions developed to solve society’s most pressing problems, from consumer electronics to drugs, renewable energy, and sustainable plastics. Over the course of 4 days, the event brought together thought leaders in academia, industry, and government to help consolidate the concept of accelerated science-research driven by artificial intelligence (AI), automation, machine learning (ML) and advanced computing-in the area of materials and molecular discovery. I recently returned from the first annual Acceleration Conference hosted by the Acceleration Consortium at the University of Toronto in Canada (August 30 th to September 2 nd, 2022 see Acceleration Consortium 1).
